AI is increasingly impacting on our daily lives, from speeding up the search for a coronavirus vaccine to predicting exam results. However, there are growing concerns about how organisations and governments can ensure they use it responsibly, so it’s not surprising that this year Gartner added ‘Responsible AI’ as a new category on its Hype Cycle for Emerging Technologies. The organisation defines this as ‘improving business and societal value, reducing risk, increasing trust and transparency and reducing bias mitigation with AI’. Gartner suggests that one of the most urgent use cases is identifying and stopping the production of deep fakes around the world.
In my view, responsible AI, while a valuable concept, is not enough to rescue AI from concerns about potential bias and discrimination. Recent issues where its use has been questioned have ranged from Amazon finding that the algorithm it was using for hiring employees was biased against women, to August’s A-level grading controversy.
AI bias: Why it happens and how companies can address it
Once trust in a technology has gone, it is extremely difficult to win back. So organisations developing and using AI need go beyond responsible AI if they want to increase the trustworthiness and transparency of their AI applications. The solution is to implement explainable AI (XAI), that is, to describe the purpose, rationale and decision-making process of the AI solution in a way that can be understood by the average person.
XAI will give the organisation deploying the solution the reassurance that decision-making is happening in a way they are comfortable with. For example, in cases when a business relies on detailed knowledge of specific individuals, such as during client onboarding or Know Your Customer (KYC) checks in the financial sector, it is crucial that it can justify every decision quickly and easily to avoid any accusations of bias.
XAI will also ensure that those subject to the decisions arising from it can be more confident that they are being treated fairly, and not subject to what the Prime Minster described somewhat colourfully as a ‘mutant algorithm’. In areas where the public is involved, it is vital that the decision-making process is totally transparent. Perceived bias may lead to AI being banned completely for a particular application, such as the recent ruling that the use of automatic facial recognition technology by South Wales Police was unlawful.
What you need to know about consumer privacy and facial recognition
From voluntary XAI to regulation
At present XAI is optional, although some enterprises are already taking steps in that direction. For example, this year Amazon announced a one-year pause in police use of its facial recognition product, while IBM decided to abandon facial recognition research altogether, citing concerns about the human rights implications.
However, regulation can only be a matter of time. In July 2020, the ICO published guidance to help organisations explain their processes, services and decisions delivered or assisted by AI to those who are affected by them. The guidance was based on public and industry engagement research carried out in conjunction with The Alan Turing Institute.
This is not a statutory code of practice under the UK Data Protection Act 2018, but advice on best practice. However, I anticipate that organisations will soon have to make their AI systems transparent from launch, demonstrating that their algorithms handle data in a way compatible with data protection legislation.
To err is human – beware the infallible algorithm
There are many techniques organisations can use to develop XAI. As well as continually teaching their system new things, they need to ensure that it is learning correct information and does not use one mistake or piece of biased information as the basis for all future analysis. Multilingual semantic searches are vital, particularly for unstructured information. They can filter out the white noise and minimise the risk of seeing the same risk or opportunity multiple times.
Organisations should also add a human element to their AI, particularly if building a watch list. If a system automatically red flags criminal convictions without scoring them for severity, a person with a speeding fine could be treated in the same way as one serving a long prison sentence. For XAI, systems should always err on the side of the positive. If a red flag is raised, the AI system should not give a flat ‘no’ but should raise an alert for checking by a human.
Five things businesses need to think about when implementing AI
Finally, even the best AI system should generate a few mistakes. Performance should be an eight out of ten, never a ten, or it becomes impossible to trust that the system is working properly. Mistakes can be addressed, and performance continually tweaked, but there will never be perfection.
AI is clearly the way of the future, as it can analyse large volumes of data much quicker than humans and help in a wide range of applications. Ensuring we develop XAI will build the confidence of those who depend on its decisions and help to grow its acceptance in new areas.
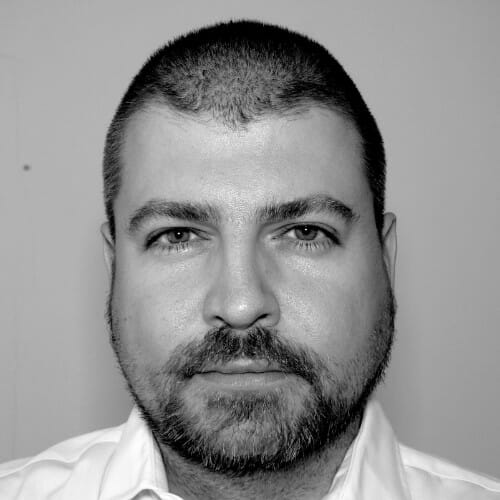