Digital twins have evolved to become all encompassing digital replicas of anything from a single object to an entire industrial process. They incorporate and synthesise multiple layers of information to become reactable in the same way as their physical counterparts. However, creating these digital twins with the highest level of detail requires reflecting the volatile nature of their properties. Supply chains can be disrupted by changing availability of resources; factory processes can be affected by temperature and pressure. Our physical world is never static, and how things change is an essential part of their makeup. There are factors involved that are time sensitive and so representing these entities to highest detail requires simulating their constant flux of change.
Cloud infrastructure has served to provide an excellent platform on which the disparate data sources can be unified and synthesised. But the centralised nature of the cloud acts as a double edged sword, as sending and analysing data to a remote location can result in latency issues. When there’s a sluggishness to this time, sensitive data, entities or processes risk being shown inaccurately. Crucial business moments can be missed, batches of product can be ruined and energy can be wasted, resulting in high costs. The advantages of cloud infrastructure are far too great to give up, but a solution to its latency problem is absolutely essential to allow digital twins to fulfill their huge potential. This is where edge computing is primed to become the next crucial piece of the puzzle.
This relationship was recently described by Gartner: “Centralised cloud was the latest swing toward centralised environments. It focused on economies of scale, agility, manageability, governance and delivery of platform services. Even so, centralising services to a cloud environment, or to other types of core infrastructure, omits some capabilities that are quite desirable. Deployments at the edge provide additional capabilities that complement the capabilities provided by the core infrastructure.”
What does the future hold for cloud and edge computing?
Edge servers process data closer to the source reducing the latency issues that occur when sending data to the cloud. This new topography removes delays created by physical distance. The processing is enabled primarily through edge network data centers. These are servers that are scattered more frequently across the world compared to cloud ones, which are often placed in more remote locations for the lower cost. Devices local to the edge servers can then connect and send and receive data without having to communicate with the further placed cloud servers.
Say, for example, you have a sensor on a valve in a factory. The sensor collects data on the pressure and temperature, which it can then send for analysis. High latency can present an issue if the valve needs to be adjusted. If the pressure is too high, and the valve is not adjusted in time, then an explosion could occur. Safety concerns such as this are key, but it also extends to optimisation of processes as well. By adjusting the valve according to real-time data, energy usage could be reduced during downtimes. By connecting to edge servers, the valve control can adjust much more quickly.
Though a huge opportunity, creating competent edge networks to support digital twins comes with its own set of challenges. Firstly, the sheer number of these kinds of IoT devices has led to a large amount of noise. That is to say, managing all the data supplied by them can be overwhelming. Much of the information varies in its relevance and value, so any edge infrastructure has to provide a system that can interpret it effectively. A network relevancy solution might address this by using spatial data structures to efficiently query which information is relevant to each client. Entities found using these queries are scheduled to be sent to the client based on metrics which correspond to the importance of that entity, enabling less important entities to be sent less frequently and reduce bandwidth.
How to control access to IoT data
IoT devices making use of edge computation have been a crucial part of building realistic digital twins. Simulation infrastructure needs, however, to include a sophisticated networking model to connect them all. Without one, systems can be prone to crashing when they have a limited number of active connections. Having an asynchronous architecture can deal with this problem effectively. It handles the actions of thousands of devices and distributed load balancing ensures the network always has enough CPU to handle large influxes. This eliminates the need of having a single thread per device and handles the control events sent by each one, forwarding them back to the simulation – the events are then reconstructed into a complete world state and then stored in a data structure.
With so much data being shared, it’s also essential that edge networks can deal with surges in demand effectively. A distributed load balancing system would ensure that the network always has enough CPU to handle large influxes without crashing. On top of this, the system needs to deal with the logging, analysis and debugging of processes within the network. A network visualiser, for instance, can provide enormous amounts of information about the connection between them. Not just latency and bandwidth, but also detailed statistics, such as the lost packets, the window sizes or the time since the last send or receive.
The importance of the edge in practical terms cannot be overstated. Speaking on edge computing’s benefits in healthcare, Weisong Shi, professor of computer science at Wayne State University, said: “By enabling edge computing, crucial data can be transmitted from the ambulance to the hospital in real time, saving time and arming emergency department teams with the knowledge they need to save lives.”
Creating the complex and dynamic digital twins of today requires reflecting the volatile nature of our modern systems. Cloud computing offers accessibility to high level processing power, and edge computing is primed to fill in the gaps.
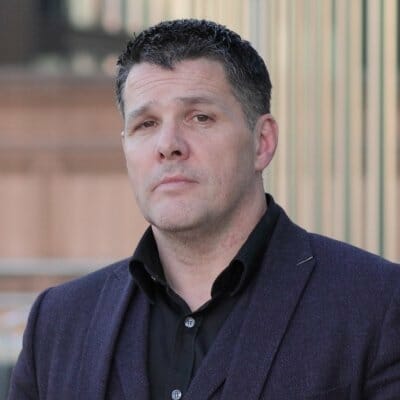