We live on the edge of an age of unlimited potential. Advances in artificial intelligence and machine learning have taken us to the point where, for the first time in human history, we can meaningfully augment the capabilities of a research team by deploying computers to do some of the work for us.
However, if we are to truly realise the possibilities offered by the ‘silicon brain’, we have to ensure that the human element of this new research tool understands the possibilities it offers and can articulate that in a meaningful way, both at the briefing stage and when applying the tool itself.
The truly ‘smart’ computer brains we have today have arrived amidst a rapid maturity of the technology. Until very recently, the capabilities of AI had initially been more often confined to areas such as gaming and rather simple chat bots, and I’m sure at the time many of us would have been cynical about the prospects of using these techniques in a scientific setting in the near future. Such has been the speed of development though that these techniques have very quickly gone from being helpful for some more ordinary tasks to becoming extremely powerful tools to drive insight and innovation.
And that perhaps explains why we have a knowledge gap when it comes to applying these tools practically.
Looking specifically at text and data mining, for example, the benefits from a high level are clear – by deploying computers to examine tens of thousands of scientific papers at once, across all disciplines, we can identify clues for further investigation and eliminate wasteful avenues of research in a fraction of the time it would take even a large team of people. The head start it will give your team in tracking down the answers or clues they’re looking for is tremendous – even before you consider any cost savings you will find as a result of those rapidly delivered insights.
And from a technical perspective, we have a good understanding of how to deploy these tools and the coding required to get the answers we’re looking for.
It is, however, between these two points that things start to slip. How exactly do you write a business case for text and data mining if you don’t know exactly what you’ll get back? For many, text and data mining could be seen as nothing more than an expensive leap of faith. In truth, it’s nothing more than a mental block.
As with any research project, it’s important we remember nothing is guaranteed in research. The same is true when asking a human to do a job rather than a computer. There are basic principles to help you narrow down your search, but you cannot guarantee that the answer you’ll get is the answer you want. A computer will just find the answers much more quickly.
Understanding that and embracing that mentality is key to overcoming one of the major human barriers to accelerated digital techniques.
Another major barrier exists – and it is perhaps even more fundamental to developing the brief. For years, computer scientists have enshrined the GIGO mantra – garbage in, garbage out. It is with good reason. Ask the right questions, you’re more likely to get the answers you want. Of equal importance is to ask the right questions of the right data – the higher quality source material, the better chance you’ll find the answers you need.
This is a basic scientific principle in itself, so it underlines the importance of not overthinking the process of developing the brief, but sitting down with someone who understands both the language of science and computing (whether that’s one person or two) to help flesh out the brief in a way that won’t just improve the results of your project from a digital perspective, but will deliver a robust framework for the human research team, who will have an understanding on what kind of clues the text and data mining project will unveil for them.
Over time, this process can become even more finely attuned and allow even greater integration between those digital techniques and scientific principles, helping us truly realise the incredible potential of AI and machine learning.
To speak to the Royal Society of Chemistry about text and data mining, visit rsc.li/tdm
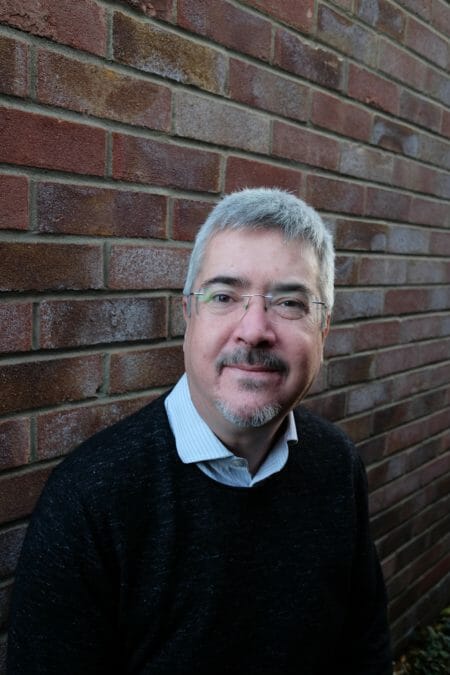