Data is being generated at unprecedented and exponential levels. By all accounts, the data economy is booming, or is it? Unlocking data in enterprise systems and gaining value from it remains a significant challenge, but it can be overcome with “hard graft”, as Murray Leach — head of infrastructure projects systems and supports at Network Rail — explained during his presentation at Big Data LDN.
Unlocking data in enterprise systems: a Network Rail case study
Network Rail is responsible for the infrastructure across the UK railway system. It spends £130 million every week on improvements for passengers and 22% of the UK’s entire infrastructure spend is delivered by Network Rail — it is Britain’s biggest builder, supporting 117,000 jobs throughout the supply chain.
The company, like so many, has amassed a huge amount of data. And by using it, “we can say what we did last month very accurately,” said Leach. “The problem is we’re not very good at using it to look into the future.”
The data journey: It’s only the beginning for digital transformation — Big Data LDN
1. Data organisation and consolidation
Responding to the challenge, Network Rail’s infrastructure projects are on a journey to become more data-centric. Part of this journey was to develop a ‘single version of the truth’ based on reports and the organisation’s large data warehouse, which impacted three key areas; business operations (using data to make business work better), report consumers (allowing project managers to look at the relevant data and make decisions) and analytics experts (to help them do their job better).
There were some common pain points that hindered this process; siloed reporting, departments, regions and executives not singing from the same hymn sheet was the problem and the process was frustrated by the inconsistent use of data.
However, the start of the data journey did glean some results; “over the last three years we have been able to work in a more controlled and automated way and improve the quality of our data from 55% to 90%. Reporting, as well, has moved from Excel and Powerpoint, and is now more specific — it takes you down to source of data,” revealed Leach.
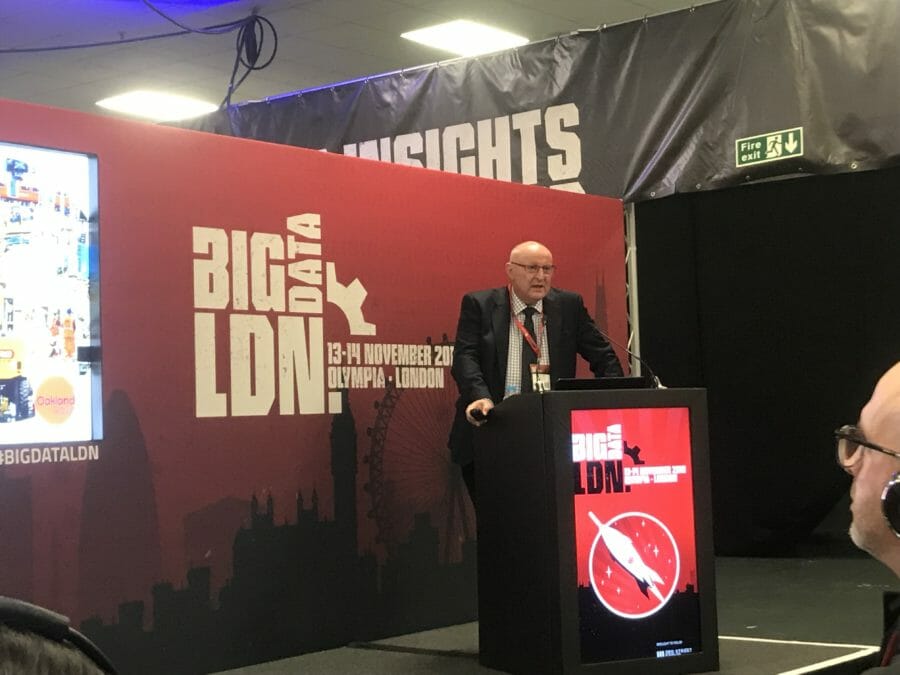
“We wanted more”
‘Simply’ consolidating Network Rail’s existing data for analysis was a start. But, “we wanted more,” continued Leach. And, by more, he meant the organisation wanted to unlock the value of data through machine learning (and artificial intelligence) and prescriptive analytics to predict the future — to understand where projects might succeed or fail, and what could be done to ensure the latter doesn’t happen.
The value of data: driving business innovation and acceleration
2. Accessing the value of enterprise data
Network Rail began the next phase of its data-centric journey by investigating it’s existing projects and “the information suggested there were kernels of good analysis,” according to Leach. From there, it moved to a proof-of-concept focusing on a machine learning approach.
“Critical outputs with significant data quality were used first; we cherry picked areas so that we could show the most immediate value, initially,” he explained.
But, even this approach encountered challenges. “The POC had to be adapted on a weekly basis to meet different group requirements.”
Network Rail has been going through a devolution process, which creates more challenges in utilising data
3. Start small and add value quickly
As with any IT project, Network Rail realised it was important to start small, ‘fail fast’ and add value quickly; create a focused value chain, use operational data, bridge the imagination gap and then combine process and analytics to add value to that chain.
It used Oakland Group for this, which helped “deliver the answers that we needed regarding the POC — cost and volume, risk data and next steps,” said Leach. “We added value to reporting, improved ad hoc queries and in terms of linking data from machine learning.”
Network Rail’s machine learning model focuses on the interrelationships between source systems — schedule, costs, risks, funding, past performance, client and supplier.
“There is a need to automate those data feeds and identify which of them we should use on different projects, such as the construction of a new station, electrification, a new tunnel, improving bridges, land management or repairing railway lines after catastrophic event. We need to be able to classify those and differentiate between green and brown fields — ML and predictive analytics can tell us about this.”
— Leach
A question then arose; “do we use large datasets for people to access or use a controlled dataset?” asked Leach.
With the severe implications for poor data management and governance, many — including Network Rail — would select the latter. This lends itself to a self-service model, where users can access certain levels of datasets. For this to work, principles need to be established; an understanding of the value chain, what the organisation has in terms of labelled data and a data dictionary that can be used to define different classifications.
“When it comes to data, getting the access rights and accessibility of it covered, is a [necessary] challenge,” confirmed Leach.
The data journey and machine learning challenge
Network Rail was used to managing data that came into reports, but when the organisation moved into predictive analytics and machine learning, it had to use normalised data in a different way. “This takes awhile to get your head around that,” explained Leach.
It also led to a number of challenges; grounding the solution in the real world, overcoming noisy data, automating manual processes and data management, locating sparse data and managing the changing technology and vendor landscape.
“It’s a question of adapting to those challenges and managing hype when using data in a bigger and better way,” he continued.
Can we automate data quality to support artificial intelligence and machine learning?
Actionable insights
Since embarking on this data journey some actionable insights have been delivered; improved ‘in Year’ forecasting, targeted assurance activity (where employees have used periodic data and data from the POC to provide assurance across a wider range of areas), process compliance and improved data quality working and unlocking reporting with advanced analytics.
Key reflections from Network Rail’s data journey
- Link process, people and tech
- Start small and add value quickly
- Pick your battles — find good data
- Tech is only part of the solution
- Proactively manage expectations
- Don’t underestimate the change (or the value)