Computer vision entered the mainstream in 2021. From NFTs and the metaverse, to traditional sectors such as agriculture and healthcare, computer vision is now the go-to technology for businesses seeking to innovate and remain competitive in the next decade.
But greater demand has resulted in bottlenecks that prevent businesses from taking full advantage of the technology. Mobius Labs has identified five issues and the steps that businesses and the wider software sector can take to overcome them.
1. Boosting data literacy will help overcome a shortfall in AI specialists
Currently, training AI and computer vision solutions require huge data sets and dedicated teams of machine learning experts, data scientists, data analysts and software engineers.
But these specialists are in short supply. Datalandscape estimated that the data skills gap of unfulfilled positions in Europe could have been as high as 500,000 in 2020, with much of the demand concentrated in the UK, France, and Germany.
As the cost of hiring continues to rise, businesses will look inwards and focus on upskilling their existing workforce. These data literate employees will be better equipped to participate in AI and computer vision projects, applying data-driven insights to improve business decision making and outcomes.
The most important skills for successful AI deployments
2. Computer visions tools will become more user friendly
As the data literate workforce expands, demand will grow for computer vision systems that are far easier to use than the complex training tools currently on offer.
In much the same way that first personal computers and then the internet transformed the workforce, this third wave of the knowledge revolution revolves around low-code tools and ‘drag and drop’ interfaces which enable employees to create and then automate processes with little prior training.
3. Businesses no longer require massive volumes of data to train algorithms
One of the key challenges of deep learning is the need for huge amounts of annotated data to train large neural networks. While this is the conventional way to train computer vision models, the latest generation of technology providers are taking an innovative approach that enables machine learning with comparatively less training data. This includes moving away from supervised learning to self-supervised and weakly supervised learnings where data availability is less of an issue.
This approach, also known as few shot learning, detects objects as well as new concepts with considerably less input data. In many cases the algorithm can be trained with as little as 20 images. This is a significant breakthrough, which means that businesses no longer need to rely on third-parties with massive computing infrastructures and data sets to create concepts that are closely tailored to the needs of consumers or business customers.
Deep tech in 2022: the future is looking artificially intelligent
4. New software helps to allay anxieties relating to privacy and personal data
Privacy remains a major concern in the AI sector. In most cases, a business must share its data assets with the AI provider via third party servers or platforms when training computer vision models. Under such arrangements there is always the risk that the third party could be hacked or even exploit valuable metadata for its own projects.
As a result, we’re seeing the rise of Privacy Enhancing Computation, which enables data to be shared between different ecosystems in order to create value, while maintaining data confidentiality. Approaches vary, but include encrypting, splitting or preprocessing sensitive data so that it can to be used without diminishing confidentiality. According to Gartner, 60% of large organisations will use some form of Privacy Enhancing Computation technology in intelligence and analytics, among other areas, by 2025.
Another approach is to remove the need to share data with a third party altogether. Some of the latest computer vision solutions enable businesses to run machine learning models on-premise, without the need for network connections. This ensures that clients have full control over their databases and maintain complete data privacy without the need to share sensitive data with providers.
5. Computer vision will be everywhere
In 2022, AI solutions will become ‘hyper present’ with the deployment of lightweight solutions on edge devices from mobile phones and laptops, to road vehicles and satellites in orbit.
To understand why this matters take the example of satellite imagery. Much of the AI processing is still done at ground level requiring the expensive download of high resolution images from space. And because there is no means of checking image quality on board, it means paying for poor quality or obscured images. Approximately 70% of content gathered from satellites is essentially worthless for this reason.
But the latest light-footprint computer vision software is power-efficient and optimised for low-latency, low-data scenarios where every ounce of space hardware is critical. This means that you can do a lot of the processing on-board and filter out useless content.
The same approach enables a press photographer to identify the most aesthetically suitable images on their laptop in a matter of seconds before sending them back to the studio. Indeed most sectors will find ways of streamlining workflows and reducing costs using computer vision at the edge.
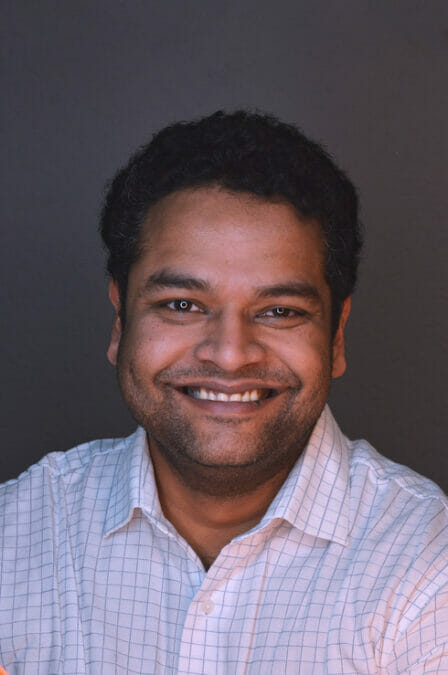