There are couple of important areas that the financial services industry is particularly interested in regarding data science.
It’s all about understanding.
The first area is to do with risk. Data science can help banks or other financial institutions adhere to compliance standards, or more specifically, credit control detection, for example.
The second area surrounds the customer. Data science can help in understanding the customer or consumer, their individual desires and pain points. All organisations, banking, B2B, they want to better understand the customer, while also exploring new avenues for business creation.
Looking at traditional banking business model, there are a lot of fintech companies trying to embrace this and change the traditional banking process.
Ryohei Fujimaki, CEO of dotData is working with a large Japanese banking customer, who is “very serious about applying data science, machine learning and AI to create new revenue sources,” he says.
These are three major areas — risk, understanding the customer and revenue creation — that banks are focusing on; using data science or machine learning to accelerate their business.
Automating data science and machine learning for business insights
It’s all about time
In any industry, “the domains are different, the business contexts are different and the data is very different,” says Fujimaki.
So, the challenge for any data science team surrounds time: it takes a lot of time to understand the data. This process typically takes two to three months for an experienced team.
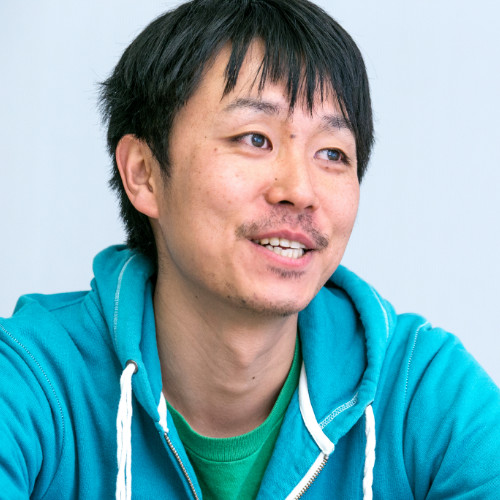
Ageing technology infrastructure
“Banks and fintech firms were early adopters of data analytics technology, but these older systems can’t handle the volume and complexity of today’s data,” says Fujimaki. “Data science technologies are known to be capable of processing massive scale of data and extracting significant patterns/insights that traditional analytics approaches would not be able to.”
Highly regulated data
“Banks work with highly regulated data and, therefore, need to ensure transparency of their data science process for data security and to certify that all financial regulations are being adhered to,” continues Fujimaki.
“Financial processes and decisions, such as determining who should be approved for a mortgage, conducting a fraud check for a credit card, or determining what the interest rate should be for a given loan, can be exponentially sped up by using AI, but the process needs to be explainable and transparent. White box data science models, where the influencing variables as well as the mathematical process to process those variables are visible and clearly explained, enable organisations to make data-driven decisions with unprecedented levels of transparency and accountability.”