At a recent Information Age roundtable in London, hosted in partnership with Informatica, tech leaders from across industries shared insights around developing data and AI strategies; and how they overcame common hurdles along their journeys.
Attendees included: Alison Choy, head of machine learning, Starling Bank; Chris Waite, head of technology, data, M&S; Sebastian Wilson, AI programme manager, HSBC; Tim Lum, head of data and insights, Virgin Atlantic; Tony McKenna, director of information technology, Gowling WLG (UK); George Zarkadakis, digital lead, Willis Towers Watson; Fergus Weldon, director of data science, Trainline; Finbarr Joy, senior advisor, Superbet; Andy Crisp, global business data strategy & stewardship lead, Dun & Bradstreet; Javier Campos, head of DataLabs UK&I EMEA, Experian; Greg Hanson, VP EMEA at Informatica and Nicholas Ismail, content editor, Information Age.
Despite attendees coming from different sectors, there was a common thread throughout the discussion: success with AI and ML starts with having a strong data foundation.
AI and data
Analytics, AI and machine learning continue to make extensive inroads into all industries presenting significant opportunities for enterprises. However, the potential for AI to improve business performance and competitiveness demands a different approach to managing the data lifecycle.
According to a consensus at the roundtable, many AI initiatives fail to launch because enterprises need insights from their data but don’t have the strategies in place to find them.
Andy Crisp, global business data strategy & stewardship lead, Dun & Bradstreet, argued that much of this problem stems from a lack of technical expertise.
“I think to succeed with data you need a really good data stewardship programme,” he said. “You need to be able to bring people in who understand the mechanics behind the data and bring context to it. I think one of the problems you see with AI is that models are being used without this knowledge and they’re going nowhere.”
Next-gen data lakes
According to Greg Hanson, VP EMEA at Informatica, the problem lies in the lack of good data governance — a problem made worse thanks to the continuing growth of data flows.
He argued to harness data, data silos must be torn down and replaced by more accessible, next-generation data lakes, which in turn, will empower more effective decision-making, more complete insights and better-informed automation.
“If you don’t provide the tools that can provision data effectively in a form that data scientists or line of business people can leverage, then they won’t be able to get that value from the data.”
The CTO role: ‘It’s about planning and business opportunities’
He added: “AI can help to make sure that that propagation of data and the lifecycle of that data is tracked, traced and made available to organisations to help manage, and prove that they are a good custodian of someone’s data.”
Organisations that can’t do this, he argued, will struggle to develop quality data products. There’s also a real risk of hindering consumer trust due to regulatory implications.
Asking the right questions
While, no doubt, AI can alleviate many of the challenges in data management, Fergus Weldon, director of data science, Trainline, said that there is a whole other issue around trying to figure out what you need from your data.
“The hardest part of my job is trying to answer the right question,” he said. “Beyond data wrangling, my team spends a lot of time actually getting people to the point where they know what they want to ask.”
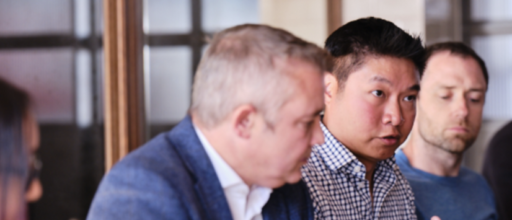
Tim Lum, head of data and insights, Virgin Atlantic, added it’s not enough to collect data and then hope AI will sort it out. Collecting data should not be the primary objective: finding a reason to collect it should be.
“Data governance is nothing without a data strategy. First and foremost, you need to figure out what the business problem is that you need to solve,” he said.
Under this conviction, much of Lum’s role at Virgin Atlantic has involved working alongside business stakeholders. For example, he recently collaborated with the Customer Experience and Cabin teams to create customer information apps for flight attendants; this way, through sharing insights and ways of working across departments, they could focus on their customers more.
He added: “From not having a data lake or warehouse to having two AI use cases, I’d say that while governance is important if you already have a lot of data, if you don’t, you need to develop a product quickly; governance can always follow.”
Echoing this view, Alison Choy, head of machine learning, Starling Bank, stated: “You have to understand your business’ needs, and what your customers want so you can decide on what products to build, and then it’s about deciding how to do it; while also making sure everybody understands the necessary steps to this goal.”
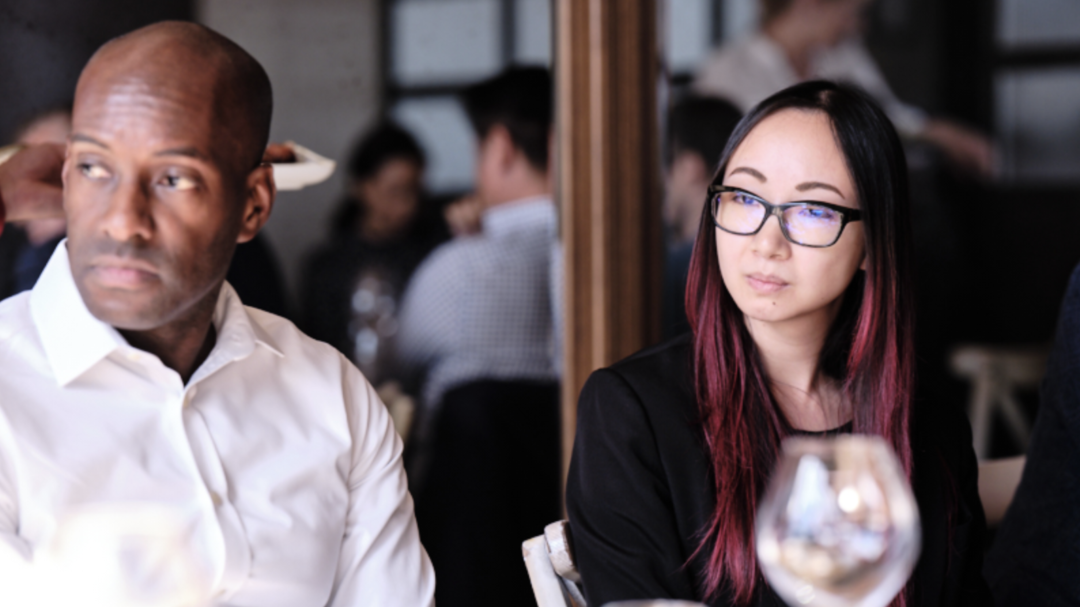
The lean CDO: why it’s time to develop a minimum viable data product
Defining data products
Tony McKenna, director of information technology, Gowling WLG (UK), made the point that this approach is also challenging. In his experience, it’s often hard to get the data team on the same page as the business analysts.
Taking up his point, Javier Campos, head of DataLabs UK&I EMEA, Experian, commented: “It appears data scientists and business analysts come from two different cultures.
“Analysts concern themselves with the business stakeholders and business impact, while many data scientists are concerned about pure data modelling.” In other words, too many data scientists lack business savviness.
This was an issue that Finbarr Joy was well aware of as a senior advisor at Superbet and was something he and his team wanted to avoid. As such they are working on dissolving siloed departments altogether.
He said: “While a bunch of specialisms are involved in getting a product to work, the data practice needs to congeal with software engineering, with product management, and the rest of the business, so they align on joined-up customer focus.
“At Superbet rather than grow separate analyst and BI teams, we are evolving multi-disciplinary product teams, and each product team has the relevant specialisms (such as ‘data’) within it.
“It’s existentially customer-driven. We must establish and enhance customer engagement — and therefore the insight underpinning it — as priority one. For us as a digital team, if our customer does not buy our proposition, as an online product, we die.”
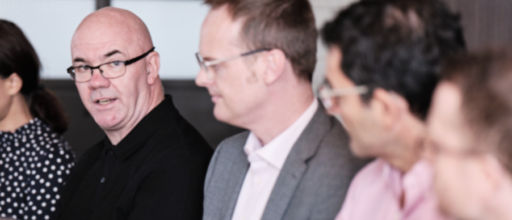
Agility and standardisation: not mutually exclusive
Whichever methodology around data management suits your business, Hanson argued that standardising the technology stack wherever possible will generally provide benefits.
He said: “A lack of data standardisation will give you a fragmentation of information which hampers organisations in their ability to drive innovation, change and agility.”
“For example, I get letters through the post from my bank (not HSBC) addressing me as Greg, Gregg, Gregory, Gregory James. This inconsistency suggests to me that many companies still have no single view of their customer and their interactions with the company as a whole.”
This has been a significant challenge for Chris Waite, head of technology, data, M&S. The retailer has a lot of data coming from disparate systems and different parts of the business.
To move ahead, M&S needed to bring those silos together so business users could innovate on ambitious projects.
Its approach to bring this federated data together was to start visualising data products via data cataloguing.
Once it had a centralised catalogue of information; it could start to bring customer data together and develop products around it.
Hanson added: “Up until now, there hasn’t really been too much investment of AI in the engineering layer. And, that’s where the big challenges around data really exist.
Artificial intelligence: Data will be the differentiator in the marketplace
“Automated governance, automated mapping of new data sources, automated insights and automated cataloguing will help.”
According to Sebastian Wilson, AI programme manager, HSBC, using AI on his firm’s data lakes have played a vital part in its road to developing AI products.
HSBC began creating its data lake back in 2017 when it pulled together data from hundreds of systems and thousands of applications; which were then tagged labelled and catalogued.
AI is used to pull insights from its data lake, helping discover commercial opportunities; as well as assessing data quality, data readiness and technical feasibility.
For many companies, trying to leap from a state of low maturity to an AI-focused model is proving to be a real challenge. Ultimately, it’s an organisation’s customers who will train their AI system, so data and AI strategies must first have a deeply vested interest in them.
Related articles
A guide to artificial intelligence in enterprise: Is it right for your business?
EU artificial intelligence guidelines will help unlock potential of AI technology
AI and data security: a help or a hindrance?
AI skills: 93% of organisations committed to AI but skills shortage is a challenge
The role of AI in cyber security
Artificial intelligence: What changed in 2018 and what to expect in 2019