Over the past 18 months, we’ve all seen how crucial the pharmaceutical industry can be for society during a public health crisis. The ability to speedily and safely discover, develop, and deliver new drugs is not just an abstract business problem, but one that the whole of society should be interested in. Improving the speed at which we can discover new drugs and compounds, whether it’s in response to a new disease or a new treatment for an existing illness, is incredibly important for all of us. And, when it comes to drug discovery, there is one technology that we know will be truly revolutionary: machine learning (ML).
Protein folding shows ML’s potential
One exciting application of ML’s potential for drug discovery that hit the headlines late last year was the challenge of protein folding, with the DeepMind team’s AlphaFold program making a huge breakthrough in the field. Protein folding – discovering the 3D structure of proteins – allows us to find out which compounds can interact with them and how, enabling us to develop new, highly specialised and effective treatments.
However, protein folding is currently very difficult – at the moment, scientists have to take a protein, dissolve it in water, crystallise it, and then diffract light through that crystal to discover the protein’s shape. This is a lengthy process and it can take weeks or months to get the data for a single protein’s structure, and then many months more to analyse that data to find out how it interacts with a variety of chemical compounds.
With hundreds of millions of proteins that would be useful to study out there, a better solution to the protein folding challenge is essential. ML is promising a potential solution: by looking at proteins that we already know the 3D structure of, models like AlphaFold can be trained to look at the raw chemical data of an unknown protein and fold it accordingly in a matter of hours or days – many times quicker than current methods.
AI use cases in healthcare for Covid-19 and beyond
The state of drug discovery
On its own, speeding up protein folding is a very exciting development. But the knock-on effects of this development are also very compelling. Currently, a lot of drug discovery requires a series of experiments in labs to determine the composition and efficacy of a drug. Labs are extremely expensive to build and maintain, so there is a limit on the number of them available – meaning that there’s a cap on the amount of teams who can discover new medicines or compounds at any one time.
ML offers the opportunity to completely change this relationship by allowing teams to do many of the vital parts of drug discovery work without entering a wet lab. Instead, that cognitive labour and time can be spent elsewhere, such as in verifying that model’s results or testing a resultant drug’s interactions with tissue cultures.
The potential of ML is not confined to protein folding either, with many tasks such as interpreting large datasets from results, making synthesis predictions for molecules and reagents, and predicting the biological properties of compounds also set to be overhauled by implementing ML models. This offers the chance for considerably faster breakthroughs by reducing the demand for lab time, and making time spent in labs far more effective. The result is that, along with potentially being far more effective at finding new compounds, ML will allow drug discovery to become much more productive than ever before.
Q&A: CTO of Healx discusses Covid-19 drug discovery and tech trends
Managing ML for drug discovery
The biggest challenge facing many pharmaceutical firms in embracing ML is not so much understanding or developing these revolutionary ML models. More so, one of the big issues lies in the logistics of deploying these models at scale. The benefits of implementing machine learning into processes are clear, the greater challenge is ensuring this technology can be deployed with the right monitoring and explainability built-in to minimise risk.
The deployment of ML models en masse for any organisation poses challenges – teams have to monitor models at scale, handle transitions between iterations of models, allocate computing and storage resources across their models, and also follow through with all the necessary regulatory and compliance concerns in their sector. For the pharmaceutical industry, this is particularly pronounced given the amount of money that is often at stake in the drug discovery process, as well as the ethical, regulatory, and social importance of robust processes for drug discovery and development.
That’s why, as we find more innovative ML models entering the fray, the importance of ML operations – MLOps – is only going to continue to grow. If we wish to see ML scale and consistently deliver on its promises for drug discovery, the role and importance of consistent and well-defined MLOps procedures and tools must grow. That way, we can ensure that ML delivers on its potential to deliver medical breakthroughs.
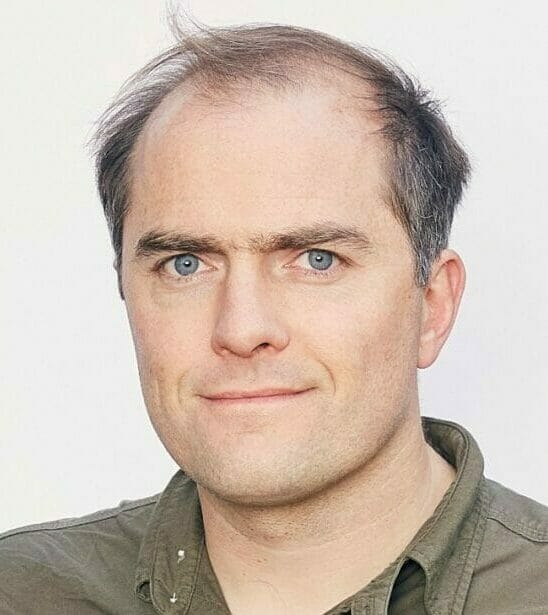
See also: Advancing precision medicine into everyday practice – John Carew, Managing Director and Health Industry Data Science Lead at Accenture, speaks to Information Age about precision medicine and its potential to impact the everyday practice of medicine