Without doubt, personalisation has huge benefits. When done well, the return on investment is significant. It’s the logical next step in the evolution of customer experience, a way of making relationships stronger and more relevant than ever before.
Recent research conducted by Wunderman Thompson Technology revealed that 35% of consumers are willing to give more of their personal data to brands if it improves the online experience. They value their data being used to provide loyalty-based discounts (55%), to be shown products tailored to their requirements (36%) and to be served offers based on their preferences (33%). When first party data is willingly exchanged, providing customers with these kinds of personalised experiences clearly adds value.
However, first party data is hard to come by, and will only be exchanged as part of a transaction or a logged-on experience. While the collection of this data is no doubt something for brands to prioritise, being able to serve up contextual and relevant content earlier in the customer journey is also crucial in creating a differentiating experience. When this goes wrong, customers are quick to respond. Our research tells us that 33% of consumers find targeted ads among their biggest frustration when dealing with brands online. This clearly shows that those ads are not, in fact, as targeted as brands would like to think.
So, where does AI come into this and how can businesses harness it to help them drive better experiences?
How AI is transforming enterprise operations and customer experience
Personalising with AI
Lookalike modelling is the practice of identifying a customer, based on their browsing history, and applying the profile of a known customer with whom they share visible traits. It’s a way of applying first party data knowledge to a segment that appears to be behaving in a similar way. AI connects the dots between existing profiles and segments of anonymous users grouped together because behavioural data shows they exhibit the same browsing traits.
This may sound fairly sophisticated, but it is not too difficult to implement. At a basic level you are just identifying users who are perhaps returning to your site for a 2nd or 3rd time and aligning them with customers who have viewed similar items, but gone on to purchase. It requires fairly high-level rules to be set, and leaves the AI to do the clever recognition work, enabling brands to reliably serve up the content that is most likely to pull those new customers further down the purchase funnel.
AI can also be applied in testing that content. In A/B testing in particular, AI can help to identity and auto-allocate successful tests as the default experience. This not only streamlines the administration of A/B tests, but also ensures agility in the deployment of them.
However, as with all technology implementations, the success of an AI implementation depends on several other factors.
Creating a strategy to tackle customer churn for a tech startup
Data and audience segmentation
Fundamental to the success of lookalike modelling and a successful AI implementation is the organisation and processing of the data-driving audience segmentation.
If a business does not understand its core customers, it has bigger issues than AI can possibly solve. Digitally mature organisations may already have customer data platforms plugged into tech stacks, which bring together data from various sources to form a consolidated 360 view of customers. Others may be looking to web analytics platforms, CRM data or commerce data to inform audience segmentation.
The bigger the organisation, the bigger the task. Larger organisations will need to prioritise the segments that deliver the most return in terms of revenue. Remember that AI can only successfully deliver personalisation results once it has been taught about a core set of known segments for targeting. Data analysts will need to create these segments, based on available data before the parameters can be set for the AI.
Why problem solving using analytics needs new thinking
Getting the underlying architecture right
It’s not just the AI engine that is key to success. The way in which your content is administered and managed will play a huge part.
In order to quickly serve up the right content, AI needs to be able to identify, retrieve and render it, and having the right content structure is key to the success to this. Content that may have traditionally lived only in the context of an authored web page doesn’t always provide the level of granularity needed to be of any use for personalised content. This is certainly true of product-based personalisation, which, in turn, requires a product-based content structure to enable personalisation engines to read individual data attributes and assemble them in real time.
Meticulous metadata is also essential to this process. Metadata is the language that AI understands; it describes the attributes of a product such as category, style and colour. Without the right metadata, personalisation engines cannot identify the right content at the right time. Fast fashion retailers, such as Asos and Boohoo, are leading the way in personalising the presentation of products to customers in this way.
Not forgetting the human element
Artificial intelligence is human taught. This is the most basic thing to remember when considering an AI implementation of any kind. To begin with, an AI engine is just a tabula rasa awaiting instruction.
So, when considering the tooling set that you’ll need to personalise successfully with AI – the bare essentials would be a content management system, asset management system and personalisation engine of some description that the AI would form a module of, or sit on top of – remember to consider the team that will drive the implementation. Do they have the skills? Have they been onboarded to really understand the technology they have at their fingertips? Technology is not a plug in; it takes a skilled team to drive it.
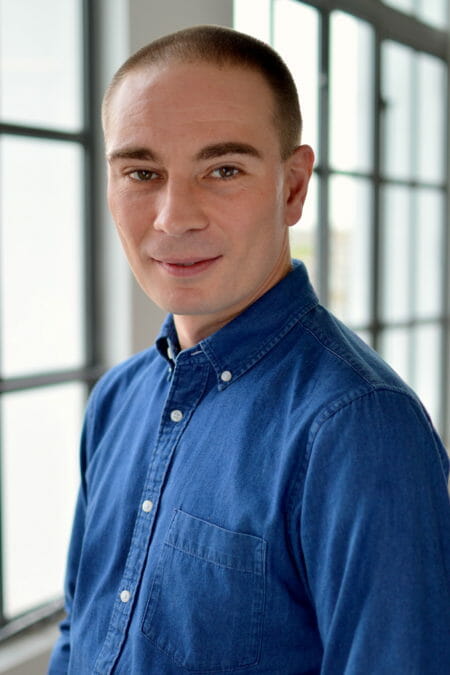