The future of data scientists
Data scientists are clever, they often come armed with PhDs, and these days, while data sits in the cloud, they often command salaries that reside above the clouds. Will it always be like that? What about the future of data scientists?
European data science salaries revealed in new report
As Claire Sporton, from CX Innovation at Confirmit, recently told Information Age: “Organisations can’t get enough data scientists to provide the amount of analytics they need. But not every team leader across contact centres, or account managers, can have a data scientist sitting shoulder to shoulder with them telling them what this data means.”
Sporton argues that “we have got to find a way to make data understandable and usable by everybody within the organisation.”
Then again, times do change. There was a time when, to produce a website, you needed to be a first class programmer. It was an expensive business. Archiving content, putting in keywords, making them easy to find; was a challenge for all but highly accomplished programmers. Then along came WordPress, and the business was transformed. It was as if the process of building websites had become democratised.
Maybe it will be like that with data science work?
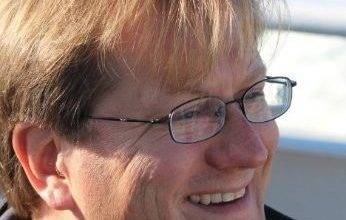
Automation of data science
Take companies like SnapLogic, which uses visual programming interfaces to do some sophisticated automation tasks and data integration tasks, without much coding. Or DataRobot, in the business of automating certain data science activities.
So, is the future of the data scientist lurking in the graveyard?
Yet, when you speak to people at DataRobot or SnapLogic, they don’t see it like that. Rather they envisage demand for data scientists going one way and that is up, but in parallel with this, increasingly more tasks, previously seen as the preserve of data scientists, may be carried out by others, or indeed, automated.
The rise of WordPress may provide a good analogy. Its success, didn’t do away with the need for HTML programmers, rather it led to a greater proliferation of professional looking websites, the need for experts still exists, to make those websites more effective. See it like an arms race: WordPress meant it was easier to build professional looking websites, but to score over rivals you still needed to buy in experts.
Simon Blunn, VP and General Manager for DataRobot, says that “the technologies that are out there take away some of that mathematical and coding requirement.” When we asked him if that meant the end of data scientists as we currently know them, he answered with a definitive ‘no!’ “We’re providing a platform that can make a data scientist way more productive, but also make a non-data scientist very productive in the world of data science without having to have that maths background.”
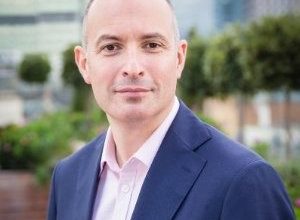
It’s the emphasis on the word ‘both’ that is crucial. Data scientists to do some of the work, non-data scientists to do less technical work. Since the demand is expanding, suggests Blunn, the market for both types is growing fast.
Greg Benson, Professor of Computer Science at the University of San Francisco and Chief Scientist at Snaplogic talks about the citizen data scientist.
“We are targeting two types of roles,” he says. “On one hand, we want to expand, we want to make a larger audience, a larger set of people who can take advantage of data science and machine learning. So that’s where the citizen data scientist or the self-service data scientist can interact visually.” However, he said that “there is also acknowledgement that there is a need for traditional data scientists too.”
Simon Blunn says “we can help data scientists automate a lot of the manual stuff that they currently do but which does not require the skills that comes with all those years of education.”
“They want to do the really hard, sexy stuff, that only they can do. I think that data science skills will still be at relative premium for a number of years. But we also want to push some of that work out to people who have not spent six or seven years in formal education trying to get to that point. So I think we’ll still see, for a number of years to come, data science skills at a relative premium but I think we’ll be able to get a lot closer to meeting demand by the use of different technologies.”
A couple of years ago, Gartner claimed that 40% of data science tasks will be automated by 2020.
Automating data science and machine learning for business insights
Citizen data scientists
Indeed, it also predicted the rise of citizen data scientists, which it defined as “a person who creates or generates models that use advanced diagnostic analytics or predictive and prescriptive capabilities, but whose primary job function is outside the field of statistics and analytics.”
So citizen data scientists it says, “bridge the gap between mainstream self-service analytics by business users and the advanced analytics techniques of data scientists.”
It also predicted that by 2019, citizen data scientists will surpass data scientists by the amount of work produced.
Well, we are now in 2019, Citizen data scientists may be becoming more prevalent, but your highly qualified data scientists are hardly going out of fashion.
It is a debate that runs across industries far away from that of data scientist. When in 2013, Frey and Osborne estimated that 47% of US jobs were in danger of automation, they obtained no shortage of headlines. Critics argued, however, that the report paid insufficient attention to how technology could free up the existing labour force to focus on the more challenging aspects of their role. Frey and Osborne, suggested critics, put too much emphasis on individual tasks that fall within a certain job role which could indeed be automated, and insufficient attention to other more important tasks, that are harder to automate.
You could perhaps copy and paste the issues that make up the debate surrounding the Frey and Osborne report into the debate on the future of data scientists. Except that is, the peculiar nature of the core work that data scientists do, the work they spent years acquiring the skills for, maybe earning themselves a PhD en route, illustrates the major issue with the Frey and Osborne predictions in a big way. Sure some of their tasks can be automated, and sure, automation can allow less specifically specialised individuals do some of their work, but the need for tasks that only they can do is growing too.
The automation first mindset
As Jeremy Achin from DataRobot said when we interviewed him last year: “If everyone on the planet became data scientists there still wouldn’t be enough.” He added: “We need trillions of AI systems, and even if you automate 80% of the work, 20% of trillions is still a massive number. It is a waste of time worrying about whether you might be fired, having lost your job to an algorithm. Instead, data scientists need what we call an automation first mindset. They need to look at their job and ask what part can be automated.”
Maybe, then, that is the key to a successful future of data scientists: the profession needs an “automation first mindset.”
Will 2019 see the automation of automation and push up salaries of data scientists?