With the growth of Artificial Intelligence (AI) applications in businesses comes the responsibility to apply these AI solutions in a smart, ethical and economically friendly way. Every new application of the technology brings its own unique challenges, so the need to constantly monitor AI use in business is vital. In light of this, here are five key things to consider when implementing, or even thinking about implementing, AI into your company.
1. Identify cases that can deliver value quickly
Return on investment (ROI) is important, but speed, however, is critical. One common mistake that many organisations make when selecting the first business challenge to solve with AI, is choosing the one that will create the biggest ROI. However, that does not necessarily yield the biggest or best result for the business as a whole. There are often much simpler problems that can generate more value, more rapidly.
How to maximise your cloud computing investment
ROI must however play a role in decision making. Under no circumstances should the cost of the solution exceed the value of its benefits, so having a good estimation of the expected ROI of any AI solution is important.
2. Always involve the business
While this seems obvious, it bears repeating: staying connected with the business throughout the lifecycle of an initiative is essential. Trade-offs and decisions will be needed along the way, and therefore it is critical to understand what the business needs to achieve; how important an issue is to them; and how quickly they need it. Understanding all of this and agreeing next steps will help to secure the necessary investment – and even increased funding if early results prove more promising than anticipated.
Managing expectations is also crucial. AI can never guarantee 100% automation or 100% accuracy. Therefore, it is very important to understand what a tolerable margin of error is for the business, and how the business plans to resolve errors or exceptions when they arise. This understanding clarifies where, how and to what extent AI can help the business and where leaders might need a backup plan.
3. Have the correct data, at the right time, ready for analysis
Any savings that an organisation might make by using AI, can easily be eroded or lost by the costs that are incurred when feeding data into the AI engine. As AI’s success is so heavily dependent on data, it has to be both high quality and trustworthy. As such, data needs to be fed to AI in real time as old data can generate insight that no longer has value. Furthermore, it should be prepared, categorised and classified in a way that makes it easy to analyse. While many digital businesses are flooded with masses of data, the truth is that it is far too often disorganised, unclassified, poor quality or out of date. So, investment in modern data platforms and data management capabilities is critical to drive successful AI initiatives.
How can leaders prepare for the business impact of automation and AI?
Alongside this, an enterprise data strategy that combines the data and allows all areas of the business to access it freely is essential. For example, a customer’s history on a consumer platform is useful for product managers to understand what features are preferred; for customer service to know what the customer has done on the platform; and for marketing to create personalised campaigns.
4. Start small, fail fast, be nimble
The phrase “first time right” rarely applies to implementing AI, and that is especially true in making predictions and forecasts. Achieving an acceptable level of accuracy could take a number of iterations and continuous course corrections. Failure, then, must happen fast in order to learn what to correct. Since the stakes are high and there is always a risk of failure, it is also important to start with a smaller problem or a subsection of a large problem. This helps to reduce the risk associated with the cost of failure. There is no shame in dropping an idea and rethinking the approach. In fact, that willingness to rethink is vital.
If the viability of a solution is in doubt, persisting with it – and, by so doing, wasting time and money – is never the right way to go. It is always advisable to change course or, in some cases, drop the idea altogether and pick a new one. Once a smaller problem is resolved and the business can see its value – and associated ROI – the solution can be scaled up to solve a bigger problem.
Maximising the value of data at SSE Energy Services
5. Measure outcomes, not outputs
IT and AI projects are inherently different. IT projects proceed with a clear idea and a set target for the desired output from day one. AI, by contrast, is mostly used in the quest to understand the unknown. It is therefore impossible to know what the output will be ahead of time. As a result, AI needs to be tuned, monitored and modified continuously over time.
Success with AI may therefore require more iterations than originally planned, and it might not deliver the same level of accuracy or automation as initially imagined. Success should therefore be judged by the degree of impact it creates and how much value that yields.
Implement AI with cause
Implementing AI ‘just for the sake of it’ is a bad idea that will cost you and your organisation precious time, effort and money. It is easy to become overwhelmed by the immense possibilities AI can bring to your organisation, just because the possible applications are almost endless. It is therefore important that businesses try to focus on what is needed and is feasible for AI in the company. Do not hesitate to try different formats, and do not be afraid of failure. AI might fail a couple of times but that is a necessary step, as AI projects ultimately will not go anywhere without the lessons learned from those failures.
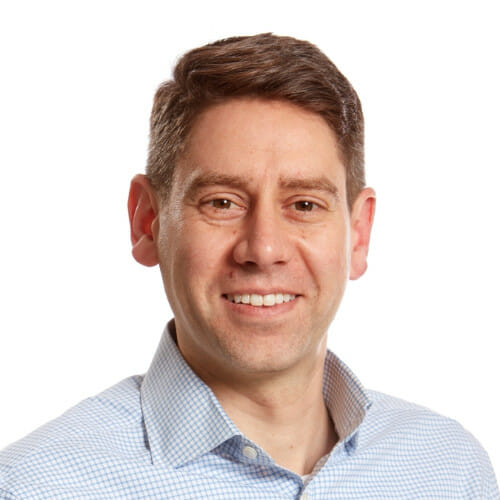