It’s safe to say that 2021 was a year like no other in terms of the innovation we saw across data science, machine learning, and AI. Many companies went far beyond weathering disruption to positively embrace it, emerging much stronger and resilient in the face of market dynamics. Today, companies recognise the benefits of putting in the hard work to operationalise data projects in order to drive meaningful change, and we’re set to see a few key AI trends that will manifest in 2022 and beyond.
1. We’ll see a shift in focus from enterprise AI to everyday AI
The ability to become a true AI enterprise will come with successfully scaling, systemising and employing robust data projects and processes at all levels of a given organisation. Everyday AI will become an organisational asset pivotal to the success of businesses of the future, regardless of the industry. The companies that succeed with everyday AI will be the ones that go beyond leveraging enterprise AI for one particular project or use case and instead will focus on scaling it out to a level that will sustain the business in the future. Scaled everyday AI companies will manage to build a foundation for data science, machine learning, and AI at an organisational level.
2. Code-free tooling is evolving rapidly, and business users are set to start delivering more value on AI than data scientists
It’s the beginning of a fundamental shift in mindset around data tooling, and we’ll continue to see a bigger breadth of people that have access to data to work with it on a day-to-day basis. Smart data ingestion, processing dates and times, clearing complex text fields, combining datasets — even creating new machine learning models — are all examples of tasks that can be done without code on many platforms. It means that the work of non-data scientists can be incorporated into data science projects in meaningful ways.
Deep tech in 2022: the future is looking artificially intelligent
3. Organisations will become better at deploying models
As they see the success of their existing data science and AI initiatives, businesses are pushing for new data science implementations in other parts of their business. As a result, we’re seeing confidence grow and more experimentation take place. This inevitably takes AI leaders out of a comfort zone where their applications of data and AI were already well understood or where they used partially digitised processes and were built on ‘better,’ more available data.
As companies evolve past this stage, new risks will gradually emerge as the next set of processes to be augmented or automated by data science are less understood. Though risk is inevitable, companies will forge ahead because they know that making the move to experiment is required in the journey to unlock the potential future of new data science and AI implementations.
4. Machine learning will be applied to enterprise IT operations and IT service issues
Historically, IT departments may have been nervous about supporting a growing number of machine learning models due to their complexity and the potential for model maintenance to become time and resource-intensive. However, as the traditional gatekeepers of all data, and with data science becoming more operationalised and democratised, IT is realising its potential to become a key business stakeholder in extracting value from machine learning. IT will begin to work hand in hand with machine learning models to solve enterprise IT operations problems and improve IT service, underpinning new avenues of value from machine learning investment.
How SMEs in e-commerce can drive value from machine learning
5. Given the growing confidence companies have with AI, we’ll expect to see the regulatory side of these implementations evolve, too
This doesn’t necessarily mean red tape, but the promises and capabilities of everyday AI will also bring changing attitudes towards how the technology is regulated and governed. We expect a bright future ahead — across all sectors — but we will also see the teams at the very forefront governed by new practices that will truly align both IT and business teams. As a result, future AI implementations will hold collaboration and systemisation at the very core — the teams responsible for data governance will have a whole range of expertise drawn from each part of the business, such as data architecture, privacy, integration, and modelling.
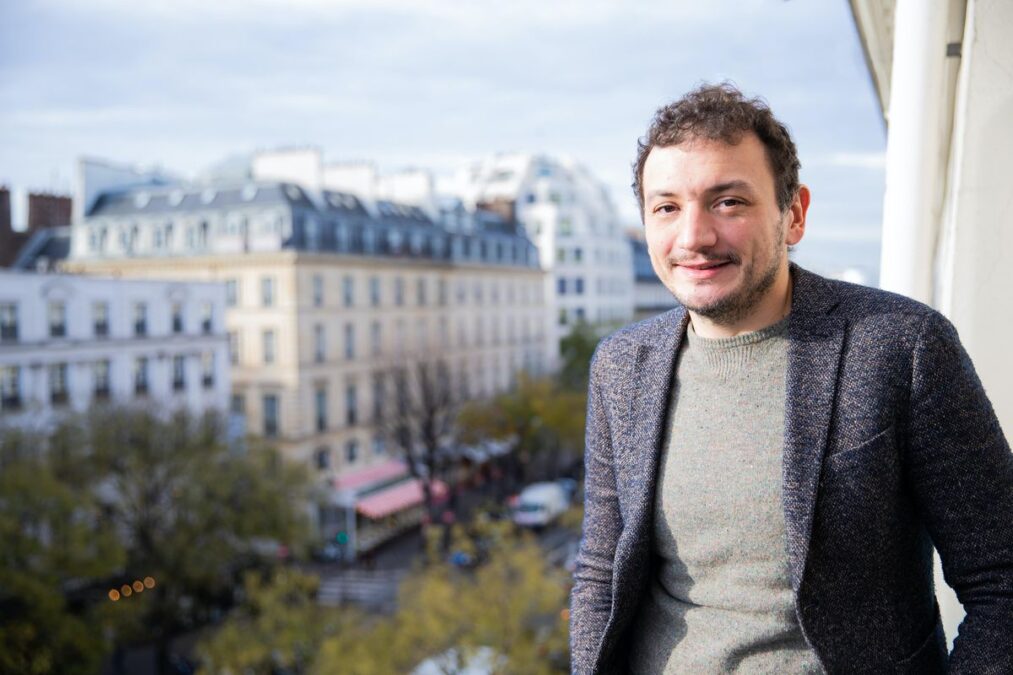