Machine vision powered by artificial intelligence (AI) is becoming considerably more efficient, and new applications are being developed at a rapid pace across a wide range of industries. This surge in popularity is one of the main driving causes for the global boom of data collection, which is expected to reach 163 zettabytes by 2025.
Machine vision and AI are gaining traction in a variety of fields, including healthcare, autonomous vehicles, manufacturing, agriculture, and security. For example:
- In healthcare, machine vision is used to quickly analyse thousands of X-rays, CAT scans, and other medical images, and save lives by prioritising patient treatment at hospital emergency rooms.
- In the automotive industry, AI-powered machine vision systems enable autonomous vehicles to identify obstacles and navigate roads safely.
- In manufacturing, machine vision plays an essential role in automatic defect detection, while the rapidly growing field of digital agriculture deploys computer vision systems to restrict or even eliminate the use of pesticides while sustainably increasing production.
What are the data management implications of AI-powered machine vision for enterprises? Most businesses are currently dealing with competing data management requirements, although the majority of data is generated at the edge, computation and storage infrastructure is centralised in a few large data centres or in the public cloud. The data must be moved and stored, which causes considerable delays and costs.
What is stopping data teams from realising the full potential of their data?
It’s a race against the clock
The majority of data collected at the edge is now moved to a central location for processing, where it is used to construct AI models. Gartner estimates that by 2025, 75% of enterprise-generated data will be created and handled outside of a typical data centre or cloud.
The process of training machine learning algorithms is dramatically hindered for firms acquiring and centralising petabytes of unstructured data – whether video, picture, or sensor data. The AI development pipeline and production model tweaking are both delayed as a result of this centralised data processing method. In an industrial setting, this could result in product faults being overlooked, causing considerable financial loss or even putting lives in peril.
Recently, distributed, decentralised architectures have become the preferred choice among businesses, resulting in most data being kept and processed at the edge to overcome the delay and latency challenges and address issues associated with data processing speeds. Deployment of edge analytics and federated machine learning technologies is bringing notable benefits while tackling the inherent security and privacy deficiencies of centralised systems.
Take, for example, a large-scale surveillance network that continuously records video. Instead of focusing on hours of film of an empty building or street, effectively training an ML model to differentiate between certain items needs the model to assess footage in which anything new is observed. Businesses may save time, bandwidth, and money by pre-analyzing data at the edge and sending only the most important footage to a central location.
While distributed designs provide a lot of advantages, they also have a lot of drawbacks. The importance of selecting and installing appropriate storage and compute infrastructure at the edge, as well as centralised management, has a substantial impact on total system efficiency and cost of ownership.
The benefits of tiered storage
Many enterprises storing vast volumes of unstructured data often rely on network-attached storage appliances or public cloud storage. However, utilising a tiered data storage architecture can help to reduce costs.
In a tiered system, content is placed on fast storage during the active period when that data is being processed and analysed, while a backup copy is stored and archived on lower-cost storage – such as tape or object storage. Lower-cost storage can go as low as approximately £37 per terabyte at scale. In many sectors – including autonomous vehicles – most data that’s collected needs to be kept indefinitely, but it’s very rarely used and can be stored at the lowest cost tier.
Many of the photos and videos gathered for AI model training should be kept indefinitely for several purposes. In sophisticated driver assistance systems and driverless vehicles, for example, the AI makes judgments based on real-time data. Businesses, on the other hand, must be able to go back and examine what happened if an issue arises months or years later. Though necessary for security, this storage comes at a high price – £2,461 per terabyte per year, to be exact. It’s simple to see how prices might skyrocket when you realise that the average autonomous test vehicle captures two gigabytes of data each hour.
Q&A: Telehouse engineering lead discusses AI benefits for data centres
What should the focus be on?
On the market, there are always new advances in unstructured data storage solutions and edge analytics. Enterprises should focus on building modular end-to-end data management systems that allow them to switch out pieces for more modern technologies as they become available to take advantage of these improvements.
Even with the greatest solutions in place, firms across various industries will have issues in successfully transporting, processing, and storing massive amounts of data recorded for machine vision. Machine vision data, also presents opportunities. In the future, photographs and videos may be utilised to build new use cases, resulting in saved data being a new cash source rather than a cost for businesses. Many organisations could employ current stored data to generate new products once more advanced analytics capabilities become accessible.
What better motivation is there to prioritise data processing and storage that is smart and efficient?
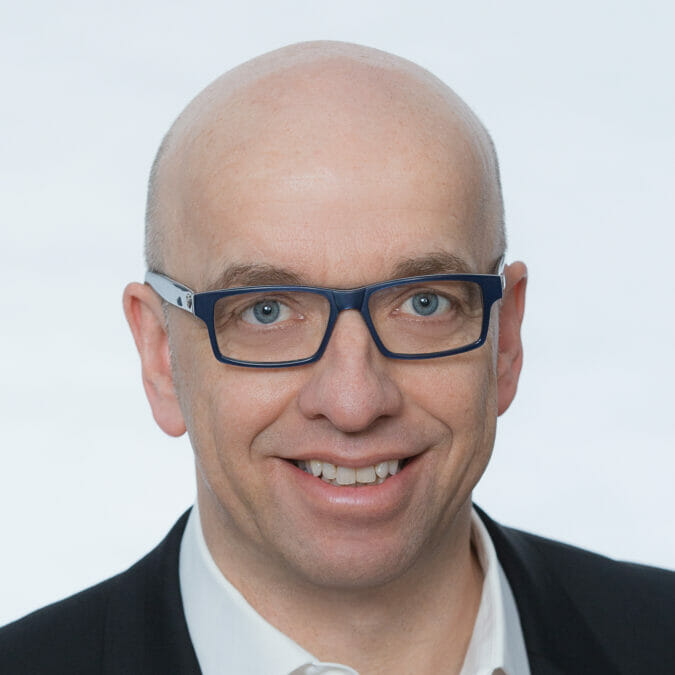