Heather Dawe, UK head of data at UST UK Data Practice, discusses how data science and artificial intelligence (AI) are driving digital transformation success across sectors
The pandemic accelerated a phenomenon that was already taking place across industry: digital transformation. Lockdowns and similar changes in our behaviours drove a massive increase in demand for online services and this demand is now unlikely to return to pre-pandemic levels.
In reaction to this, businesses of all shapes and sizes are striving to make their existing business models increasingly automated and digital-first in a bid to avoid being disrupted. They are also disrupting themselves, changing their ways of working using data and technology in a bid to improve their products and services, remain competitive and create new markets.
Central to successful digital transformation is the effective use of data. The personalisation of online services is a key example of how data is used to generate AI that achieves this. Such initiatives frequently strive to place the user or customer in greater control, catering to them by predicting their requirements and subsequently personalising the service to them. Data is used to train machine learning models underpinning an AI service. The AI predicts the user requirements and configures the service to these requirements.
The desire to accelerate digital transformation programmes is a large contributor to the increased demand for data scientists and data science skills within industry. In 2019 the Royal Society reported a threefold increase in demand over five years. Subsequent year-on-year increases in demand have been at least 30 per cent.
Data science and AI across industry sectors
So, what are all these data scientists doing and where are they doing it? At UST I work with Clients from a variety of industry sectors. They typically fall within the retail, asset management, banking & financial services and insurance (BFSI), manufacturing and automotive domains.
One of the fascinating things about this from a data perspective is the variation in which these sectors have so far adopted and utilised advanced analytics and AI. Asset managers for example generally use quite different forms of analysis and machine learning models than retailers.
There are also similarities across sectors. Customer personalisation is a common requirement and analytical pattern within a number of sectors including retail, insurance and banking. Supply chain optimisation has significant applications across retail, manufacturing and the automotive industry.
AI in asset management
From our perspective, asset management is among our most advanced spaces for use of analytics, machine learning and AI. In addition, they are increasingly successful in implementing analytics and AI services – processes that have been recognised by Gartner as difficult to achieve.
Asset management as a discipline has used data and analytics to inform investment strategies for a long time. As data scientists within these companies become increasingly adept with programming languages such as Python and R; sophisticated in the data science methodologies they employ; and ambitious about data they use to develop and test strategies, this trend is set to continue.
Retail
The retail sector, unsurprisingly, is relatively advanced when it comes to using machine learning and AI. Data-driven loyalty and customer reward services were introduced back in the early 2000s, and since then — due in a large part to increasing competition — data innovation for customer personalisation, among other use cases, has been significant.
While the retail world can be complex, we are seeing significant growth opportunities where advanced analytics and AI across supply chain management can be implemented, along with omnichannel infrastructure.
Banking and financial services
Innovation within banking and financial services is being largely driven by online fintechs and open banking.
Given their nature of growth – from startups to more established SMEs and beyond, the challenger banks have the advantage of data-driven approaches from the get-go. Unlike larger, incumbent retail banks, they do not carry legacy systems or years of technical debt. Challenger institutions have been quick to realise the benefits of innovating with data and AI.
Open banking has brought with it a greater innovation opportunities for using banking data. These include developing new products and services, delivered as apps straight to mobile devices.
As a result, there is pressure on the long-established banks to evolve, adapting to meet the requirements of customers who expect information and services to be immediately accessible 24/7. The large retail banks are innovating with their data more than ever before.
Insurance
Retail insurers are yet to face the same pressures to evolve in the market as retail banking. But this doesn’t mean these requirements are not present. For example, the growing gig economy is driving the need for small business insurers to supply personal indemnity insurance weekly, daily, or even hourly, going beyond the current standard yearly premiums offered by larger insurance companies. These incumbent insurers have similar legacy systems and technical debt as the large banks, and as a result response is slower to the changing needs and expectations of customers.
New products and services in the insurance space are commonly being developed by startups and SMEs. These often require deployment of predictive analytics due to costs of insurance products and services being underpinned by the relative risk they carry. Like the challenger banks, insurance startups and SMEs are less encumbered by technical debt than their larger competitors, cutting time-to-market.
Realising this trend, larger incumbent insurers are meeting the challenge of innovation in the same ways as the large banks: through acquisition and data-driven product and service development.
Staying competitive requires harnessing AI
Prior to the pandemic, data science and the associated development of AI-driven services was probably close to the bottom of the hype curve. These are complex subjects, difficult to scale and gain a return on investment. While the complexity remains, the past few years have seen an increasing maturity within enterprises to be able to productionise and exploit AI to their commercial benefit. In my view, we are the tip of the iceberg — the pandemic has significantly accelerated the pace of development, and there are many more digital transformation programmes on the way to yielding streamlined and improved services. Chief experience officers (CXOs) across industries have realised that there is no going back, investing in the data strategies and associated data development to put them in a position to remain competitive with their peers, as well as developing new digital products and services.
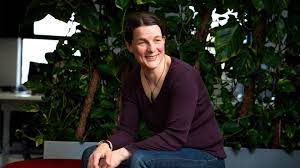
Related:
5G and AI use cases – how 5G lifts artificial intelligence — 5G will unleash the potential of AI, says Michael Baxter. But how will AI and 5G most affect our everyday business lives? What are 5G and AI use cases?
Accommodating the influx of data in the metaverse — Guido Meardi, CEO and co-founder of V-Nova, discusses how metaverse stakeholders can accommodate the pending influx of data to drive value.