Financial organisations need to continually review their game plan in order to combat the ever-changing face of digital fraud. The approach of simply monitoring for unusual transactions is no longer sufficient as a strategy. This frequently results in false positives, which in turn require large operations teams to carry out manual reviews, and the real fraud cases can often slip through the net. Introducing complicated authentication flows, however, tends to put off customers, who are accustomed to the ease and simplicity of online banking.
It is the very boom in online services, including online banking, that has led to the emergence of new types of fraud. So-called account takeovers represent a form of digital identity theft that allows criminals to access another person’s online accounts. They may then be able to purchase products and services or transfer money by using that person’s account information. In this day and age, accessing someone’s account online with a stolen set of credentials is the new approach to robbing a bank – without the danger of getting caught or inherent risks of doing this at gunpoint.
Banks are therefore doing well to invest in more sophisticated digital defences that are focused on analysing digital intelligence. These rich datasets are built up over time, establishing a digital signature for customers as they access online banking services. This technique enables banks to effectively link digital identities to accounts and determine if the latter are being accessed by a fraudster rather than the genuine customer. As a result, this type of ‘third-party’ account takeover fraud can generally be contained.
Yet, fraud continually evolves. Initially, cybercriminals could shift to target banks with weaker defences, but as the use of digital intelligence becomes common place, fraudsters have needed to adapt their attack methods.
How to use process data mining to improve DevOps
The end users themselves turn out to be the weakest link in what are classified as ‘social engineering’ attacks. Social engineering fraud can refer to a variety of different scams, but a classic example involves the criminal phoning up the customer and convincing them to move some or all of their money to a different account. One such scam involves the criminal on the phone claiming to be the bank, who is phoning up the customer to ‘warn’ them that their account has compromised and that they need to move their money to a new account. The criminal helpfully walks them through the process of transferring their money to an account that the fraudster has access to.
Alas, social engineering attacks are very difficult to stop. Although existing fraud detection systems have that wealth of digital intelligence data at their disposal, the fraud models are generally focused on looking for evidence of the ‘third party’ fraud discussed earlier – in other words, they are looking for unusual attempts to access online accounts. But in these cases, since it is actually the real customer accessing the account and moving money, fraud detection systems don’t flag this behaviour as a risk.
Against this background, financial organisations decided to take another look at the digital intelligence data they have, rooting for clues they can use to identify these new kinds of attack. As it stands, there are some indicators in digital intelligence data that can help detect certain types of scams. Timing is one of them. Say, the fraudster was successful and convinced their victim to transfer money to another account. The individual’s interaction with online banking, for example the time it takes them to login and actually move the money, can be slightly different from how they normally behave.
Existing data reveals these subtleties only to some extent. This is where a new area of digital intelligence focused on ‘behavioural biometrics’ comes in. It helps analysts to get down to the nitty-gritty by specifically looking at event timings and interactions with the keyboard, mouse or mobile touch screen, to look for these behavioural anomalies. Unfortunately, this approach is not perfect, and returns banks to the dilemma of both a high number of false positives in the data and the need for larger operations teams for manual review.
Accounts payable automation: Fighting fraud one payment at a time
So, what else can be done? For a scam to be successful, cybercriminals must have accounts available into which the victims can transfer their money. These accounts are known as mule accounts. Once the money lands in these mule accounts, it is rapidly transferred to other mule accounts and eventually cashed out in some way, be it in the form of credit card purchases or cash machine withdrawals.
Although banks are generally on the lookout for mule accounts, it is difficult for existing fraud models to identify them. Typically, the mules themselves are moving the money between their accounts, so this is not flagged as unusual. In addition, analysis of these accounts will always be a lower priority for the banks than real customers who are reporting actual fraud losses from their accounts.
Mule accounts tend to form complex networks through which funds are transferred, often traversing multiple banks and crossing geographical borders. It is very difficult for a single bank to identify these networks just by backtracking money transfers and linking accounts together. More often than not, such investigations only result in mule accounts being closed long after the funds have gone.
But in the last twelve months banks have begun to revisit the digital intelligence they have to look for alternative ways to identify mule networks. This approach has actually proved to be very successful – it turned out that links between mule accounts have been part of the digital identity data all along. New models focused on detecting these links in real time are now essential for blocking significant amounts of funds that are channelled through mule networks.
Mule networks have a wider usage for various criminal activities including general money laundering and even potentially terrorist financing. The importance of putting a stop to mule accounts must therefore not be underestimated. New approaches based on advanced behavioural analytics of global digital intelligence may be the answer.
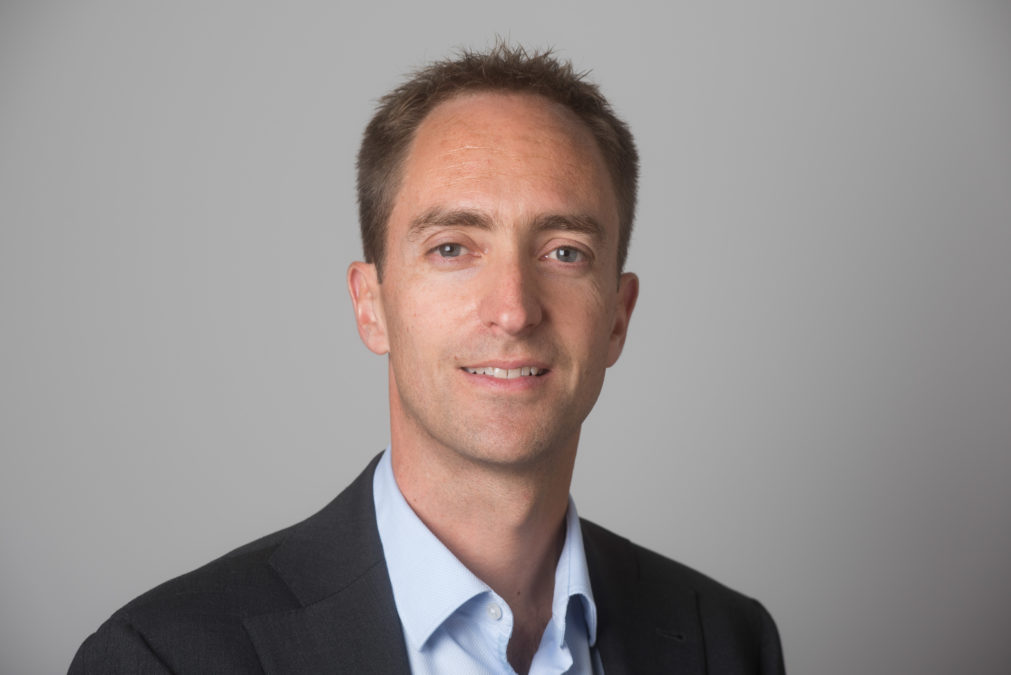